Why I Left McKinsey as a Data Scientist
Things you should consider before starting as a data science consultant
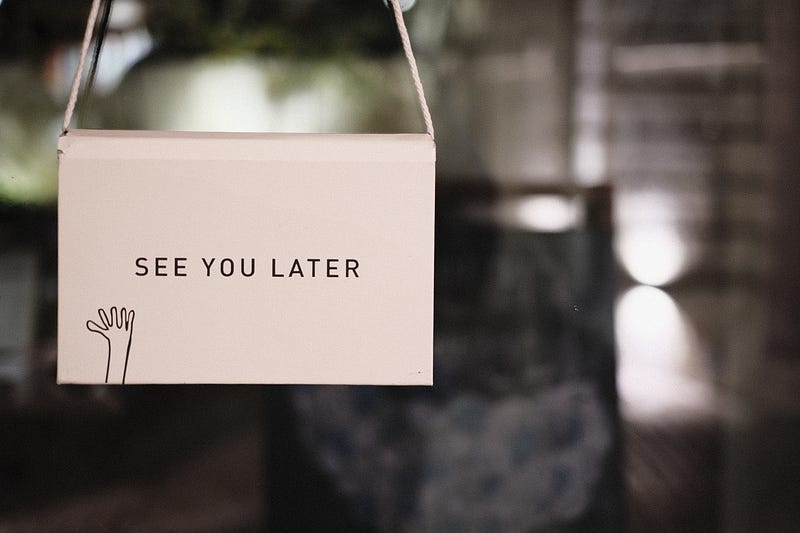
Things you should consider before starting as a data science consultant
In my previous articles about McKinsey, I talked about why I decided to join McKinsey as a data science consultant and why I think it’s a great career move for aspiring data scientists; I have also talked about valuable lessons I have learned from being a data science consultant.
Given the prestige of the company and the great things I mentioned in those articles, it was a surprise to a lot of people that I chose to leave after two years. In this article, I want to share what drove my decision to leave and what you should know and take into consideration if you are also thinking about joining consulting as a data scientist.
And just to steal the thunder of the negative voices that appear every single time I mention McKinsey in my articles — the reasons I will be mentioning here are solely out of the consideration for personal and career development; as for debates about “whether the firm is ethical”, “whether consultants are useful for companies” etc., everyone is entitled to their own opinion and those are out of the scope of my discussion here.
So with that out of the way, let’s talk about why I left:
It’s hard to feel the long-term impact of your work as a DS
You probably hear A LOT OF consultants saying this is THE reason why they have left consulting. I used to think it’s just a convenient reason people use in interviews but it turned out to be one of the main reasons that drove me to leave in the end.
This is partially determined by the nature of consultancy. Consultants provide expertise and advice in certain fields to companies. At the end of each engagement, the proposed solution (usually a model or an analysis for data science engagements) will be wrapped in a bow and handed to the client to implement, test and/or maintain. Imagine you built a demand-forecasting model for a client as a data science consultant: You can assess the model’s performance using historic data before handing it off to the client, and if the partner manages to convince the client to extend the project to test the model, you might get several weeks worth of new data to assess impact. But most likely you won’t know its performance after it’s officially deployed by the client (or if it will be deployed and used at all), let alone its continued impact several years down the road.
Until this day, it’s still a debate whether the implementation phase should be part of consulting firms’ scope. Some people believe if consultants start to implement the solutions proposed, they are taking on the role of managers while others think proposal of solutions without implementation is a waste of time and money. McKinsey started taking implementation more and more seriously during my tenure; but at the current stage of the industry, aspiring data science consultants should not get their hopes up that they will see their models in production and be able to observe longer-term impact.
Not many chances to work with cutting-edge DS concepts
Companies hire consultants for the expertise they DON’T have. So naturally, most clients that work with consultants for analytics needs are not the most advanced on the data science front. As a data science consultant, you are most likely NOT going to work on building a recommendation engine for Amazon or search optimization for Google.
There are projects here and there focusing on relatively cutting-edge techniques such as dynamic pricing, but the majority of the engagements will be utilizing simpler methods. Not to mention that clients who are just starting out on developing their analytics practice usually have more distrust for black-box models and prefer interpretability over it. As a data science consultant, you will have more opportunity to learn how to explain analytics concepts to non-analytical audiences (which in my opinion is an extremely valuable skill), but less opportunity to implement the newest practice in the DS field.
This is a more relevant concern for some than for others. If your interest as a data scientist lies in machine learning, AI and/or other advanced analytics areas, it’s worth keeping this in mind before deciding which projects you want to work on in consulting, or if consulting is the right industry to enter at all. However, if you are more interested in solving real-world business problems through data and/or modeling, consulting is the perfect place to start learning and practicing those skills.
Scalability is not always top of mind and it’s hard to think about/take on long-term initiatives
Since consulting engagements are usually short-term, even squeezed for time occasionally, clients expect to see deliverables within weeks. Due to short timelines and the fact that most times consultants are not held accountable for the sustainability of the deliverables after the handoff, efficiency usually takes priority over scalability when it comes to choosing approaches. A lot of the analytics deliverables, models or analyses end up being more of a “proof of concept” or “prototype” than a real fully-fledged product.
Ever since working in the tech industry, especially as a manager, I have learned the importance of long-term initiatives. These type of projects are usually initiated by managers and leaders who elevate themselves from their teams’ immediate day-to-day scope and proactively address gaps in realizing the whole company’s vision. Consultants hardly ever need to think about long-term initiatives because they are hired to solve a (or a set of) problem(s); so the nature of the work is reactive instead of proactive and the scope of the work is normally pre-defined. Imagine you are hired to figure out how to place a piece of the puzzle, but you don’t have a say in the how the rest of the puzzle can be moved around; it can get frustrating and you don’t get to develop an understanding of the rest of the puzzle and thus never develop the skillset to move those pieces around.
The long hours are real and the traveling can get frustrating
The final reason is more on the personal level than on the professional level. Due the nature of the work and the industry (like lawyers, consultants bill their clients by the hour), consulting is not known for a great work-life balance. Churning out analyses/decks until midnight is the norm for most weekdays.
On top of the long hours, pre-pandemic, consultants need to travel to a different city from Monday to Thursday for most projects. You might be thinking to yourself, “that sounds exciting, I get to visit different cities!”, and that’s what I thought, too, at the beginning. However, the excitement of travel for work wears off pretty quickly, especially since you have limited time to explore the city and spend most nights working late in similar-looking business hotels.
Summary:
Like I mentioned in my previous article about why I decided to join McKinsey as a data scientist, even though consulting is a great place to start your career as an aspiring data scientist, it might not be for everyone. Things to keep in mind about being a data scientist in consulting:
Consulting might be a better fit for you if you are more of an “explorer” than a “builder” because for most projects, you will hand off the prototype to clients and won’t see it grow
In general, you will acquire more data story-telling skills than cutting-edge modeling skills
You will get more opportunities to engage in efficient problem-solving with short-term analytics projects; but less chance to do long-term planning for robust analytics ones
Interested in reading more about data science career? Here are some articles that you might be interested in:
Why I Joined McKinsey as a Data Scientist
Should you ever be a data science consultant despite the 80-hour work weeks?towardsdatascience.com
5 Lessons McKinsey Taught Me That Will Make You a Better Data Scientist
towardsdatascience.com
5 Mistakes I Wish I Had Avoided in My Data Science Career
I learned these lessons the hard way so you don’t have totowardsdatascience.com